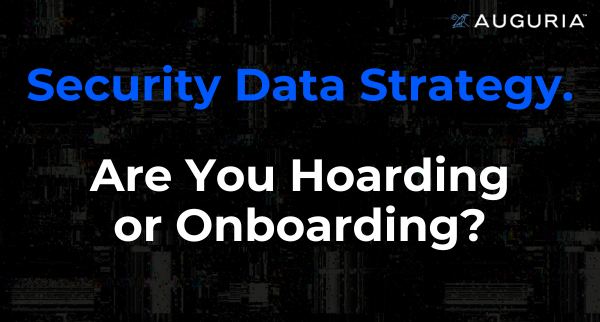
Not so long ago, there was a time when not having sufficient data at hand was one of the first challenges incident response teams faced. But in the case of “be careful what you wish for," today we have a situation where we have too much data to store and make sense of, leading to data overload and slowing down the entire incident response and security operations (SecOps) process as a consequence. As an industry, we overcorrected the problem and have instead fallen into the LASSA trap, “Log All, Store All, SIEM All" and Think Later. As a consequence, we are now hitting the law of diminishing marginal utility, and with every additional increase in security data, the speed of security operations might actually be reduced.
At the same time, the cost of security operations is increasing: licensing fees, data storage, egress and compute. Its not just the licensing cost but more human resources are required to drive all of it all add to the overall total cost of ownership (TCO). This increased complexity increases the TTV, the time taken to realize any sort of value. Despite all the efforts, we continue to fail at better, cost-effective security.
Inexorable Data Growth requires a new Mindset
The rate of data generation continues to climb. The average number of devices and apps per person is still rising, and businesses are on a continuous transition to digital, so we are at the edge of a slippery slope. As data grows, so does FOMO, or in this case, we’ll call it fear of the unknown and Fear of Missed Detection (FOMD). SOCs usually have FOMD and therefore often tend to store and hoard everything in SIEM/XDR that is available rather than storing only what is required for detection logic and security. This preference for storing more data is only natural because each device, process, and application represents a potential attack vector that needs to be protected. But whether these assets aid in high-fidelity attack detection, that's a question one should ask.
The underlying architecture of SIEM and security analytics platforms has difficulty adding value to today’s data volumes. Most of them are built on tech from a bygone era. They are typically built for data querying rather than real-time processing, and that adds to the slowness and complexity because of the sheer volume of data with mixed relevance, redundancy, and quality. It is very commonly said, “Security is a Data Problem,” which is 100% true, but if we are being more precise, it has gradually become a Data Management problem.
Absence of Security Data Strategy
SIEM/XDR vendors often recommend the ‘All you can Ingest’ approach, and they are incentivized to, as the licensing model is based on volume of data ingested rather than the value it adds. Hence, not too much attention is being paid to improving the quality of the data by enriching and preprocessing the data to make it more meaningful when ingested, leaving SOC teams to make up for the shortfall. Don’t forget, not all data is created equal, and it varies in quality, value, and usefulness.
Cleaning the data and getting it into shape is only the beginning; cost concerns and complexity associated with security operations are common concerns.
- With split architecture models of SIEMs, where users can bring their own data warehouse, such as Snowflake, computation power and cost become a major concern.
- Adding computationally expensive detections and models when each one adds cost without a high degree of fidelity and reliability of detection is an expensive exercise in futility.
Thus, increasing the value of data and increasing actionability by preprocessing the data is not just an imperative to augment human analysts to respond faster and more effectively, but also a linchpin for efficient analytics and AI.
So in the future, CISOs should not only aim for visibility but also actionability. It may seem easier to gain greater visibility with an "All you can dump” approach. In the pursuit of security, SOC analysts often forget the basic rule: security is about priority, so prioritize the data that needs to be ingested into the SIEM for priority detections.
However, without prioritization of data and “All you can dump” security operations ends up being a lot more complex, and in the messy reality of detecting evasive threats, it quickly overwhelms signals with noise. Hence, it is imperative that CISOs go back to the drawing board on how you look at the data that you are ingesting into your SIEM/XDR.
All the data generated:
|
|
It is never the volume of data that matters, but the data that yields results, ideally an actionable outcome. Hence, an effective data strategy is an imperative for organizations to maximize the yield, outcome, and ROI of the data that is being ingested, stored, and analyzed.
The use case should define the data that it needs, the compute power it needs, the longevity in storage it needs, how fast it is needed, how often it is needed, and what format it is needed in. The ‘All you can dump’ approach only leads to data overload, increased cost, and complexity with increased frustration.
In cybersecurity, a GPA (general purpose approach) can prove to be more dangerous; hence, it not only needs a data strategy but a “security data strategy." A ‘Security Data Strategy’ that is crafted according to the security maturity, SOC operating model, budget, compliance requirements, privacy regulations, and many such factors.
In the next article, we will take a more detailed look at what an effective security data strategy for an organization should look like and the outcomes it would yield.
The Time is Now:
“To build a ‘Security Data Strategy’ suited for your own organization to find the right optimum balance among cost, performance and scale.”
This site is protected by reCAPTCHA Enterprise and the Google Privacy Policy and Terms of Service apply.